
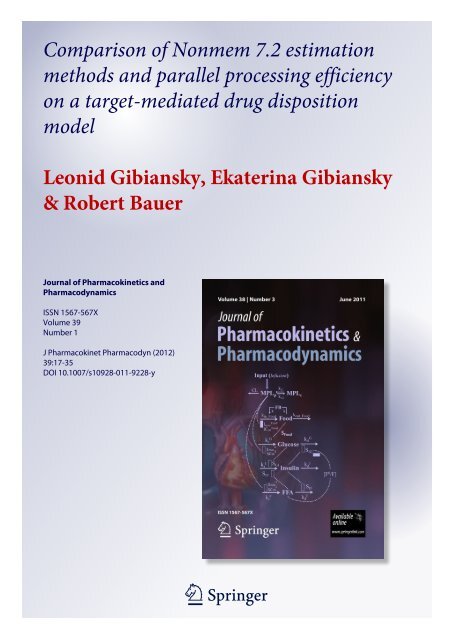
#Importance sampling with prior nonmem full
For simultaneous estimation of the full model, the ratio of estimated to true values for structural model parameters (median over 45 parameters) was 1.01 for means and 0.99 for between-subject variances for frequent sampling and 1.02 for means and 1.02 for variances for sparse sampling. Datasets comprised three dose levels with 16 subjects each. We set initial estimates to 0.1 or 10 times the true value and evaluated 30 bootstrap replicates with frequent or sparse sampling. Simpler models comprising 15 parameters were estimated using three of the ten dependent variables. We assessed bias, imprecision and robustness of this algorithm in S-ADAPT for mechanistic models with up to 45 simultaneously estimated structural parameters, 14 differential equations, and 10 dependent variables (one drug concentration and nine pharmacodynamic effects). Our objectives were to evaluate an importance sampling version of the MC-PEM algorithm for mechanistic models and to qualify the default estimation settings in SADAPT-TRAN. To build the sample, look at the target population and choose every fifth, tenth, or twentieth name, based upon the needs of the sample size.The Monte Carlo Parametric Expectation Maximization (MC-PEM) algorithm can approximate the true log-likelihood as precisely as needed and is efficiently parallelizable.

Stratified sampling: Stratified sampling is when the researcher defines the types of individuals in the population based on specific criteria for the study.Random sampling: Random sampling is when all individuals in a population have an equal chance of being selected.The three main types of quantitative sampling are: The goal is to test a theory and support or reject it. Researchers establish general laws of behavior found in different contexts and settings. Quantitative research is used to categorize, rank, and measure numerical data. Snowball sampling: The participants in the study refer other individuals who fit the traits required for the study, to the researcher.Selection of participants that meet certain traits like gender, age, health, etc. Quota sampling: The researcher establishes participant quotas before forming a sample.Purposive sampling: Pre-selected criteria related to research hypothesis determines the participants for research, for example, a study on cancer rates for individuals who live near a nuclear power station.There are three main types of qualitative sampling: This helps to interpret and measure how these elements affect humans or other living beings.

In Qualitative research, non-numerical data is used to study elements in their natural settings. However, one works better than others depending on research needs. When the researcher desires to choose members selectively,non-probability sampling is considered. Both sampling techniques are frequently utilized. Non-probability Sampling is a method wherein each member of the population does not have an equal chance of being selected. Probability Sampling is a method wherein each member of the population has the same probability of being a part of the sample. This sample might be a group of coal workers in one city. So, the researcher would need to narrow down the population and build a sample to collect data. However, it would be impossible to study a large population of coal workers. Illustration of the importance of sampling:Ī researcher might want to study the adverse health effects associated with working in a coal mine. The more representative the sample of thepopulation, the more confident the researcher can be in the quality of the results. The sample size is the number of individuals in a sample. A population is a group of individuals that share common connections. In research design, population and sampling are two important terms.
